- Published on
Unlocking the Power of Machine Learning A Comprehensive Guide
- Authors
- Name
- Adil ABBADI
Introduction
Machine learning has revolutionized the way computers interact with humans and make predictions about the future. From self-driving cars to personalized product recommendations, machine learning is transforming industries and revolutionizing the way we live. In this article, we'll explore the fundamentals of machine learning, types of machine learning, and some real-world applications that will leave you in awe.
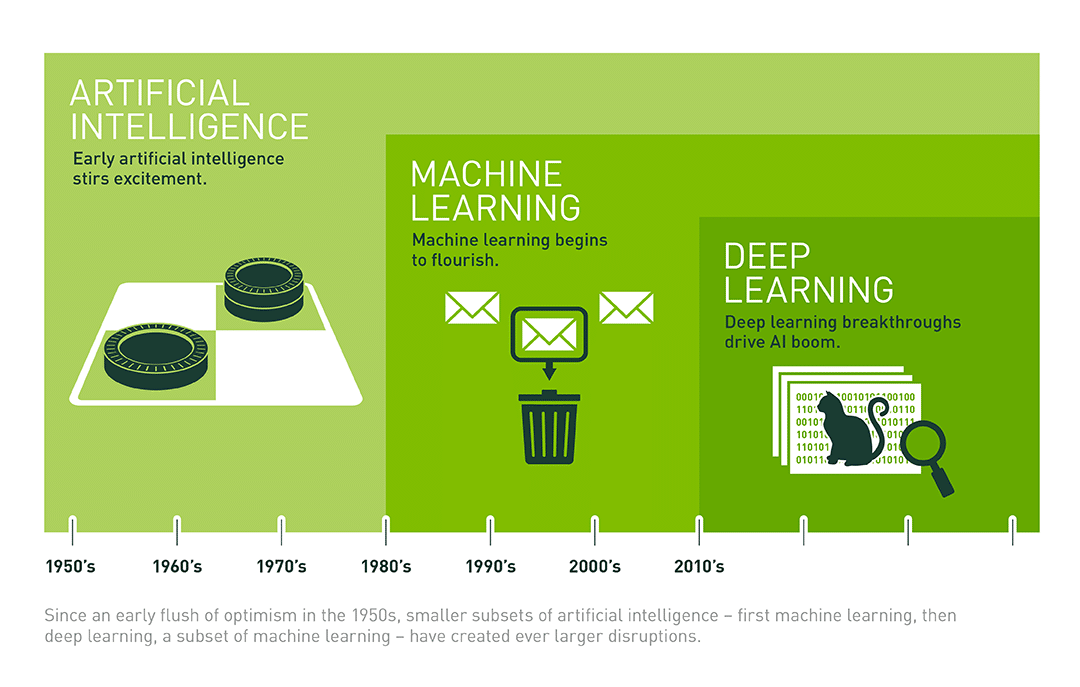
- What is Machine Learning?
- Types of Machine Learning
- Applications of Machine Learning
- Conclusion
- Start Building Your Machine Learning Project Today!
What is Machine Learning?
Machine learning is a subfield of artificial intelligence that enables computers to learn from data and improve their performance over time. The core idea is to train an algorithm on a dataset and allow it to make predictions or decisions based on new, unseen data.
# Importing scikit-learn library
from sklearn.datasets import load_iris
from sklearn.linear_model import LogisticRegression
# Loading the iris dataset
iris = load_iris()
X = iris.data
y = iris.target
# Training the model
log_reg = LogisticRegression()
log_reg.fit(X, y)
# Making predictions
predictions = log_reg.predict(X)
Types of Machine Learning
There are three primary types of machine learning: supervised learning, unsupervised learning, and reinforcement learning.
Supervised Learning
In supervised learning, the algorithm is trained on labeled data, where each example is accompanied by a target or response variable. The algorithm learns the mapping between input data and output labels, enabling it to make accurate predictions on new, unseen data.
# Supervised learning example using scikit-learn
from sklearn.datasets import load_boston
from sklearn.linear_model import LinearRegression
# Loading the Boston housing dataset
boston = load_boston()
X = boston.data
y = boston.target
# Training the model
lr = LinearRegression()
lr.fit(X, y)
# Making predictions
predictions = lr.predict(X)
Unsupervised Learning
Unsupervised learning involves training an algorithm on unlabeled data, with the goal of discovering patterns, relationships, or structure in the data. Clustering, dimensionality reduction, and density estimation are popular unsupervised learning techniques.
# Unsupervised learning example using K-means clustering
from sklearn.cluster import KMeans
from sklearn.datasets import load_iris
# Loading the iris dataset
iris = load_iris()
X = iris.data
# Training the K-means clustering model
kmeans = KMeans(n_clusters=3)
kmeans.fit(X)
# Predicting cluster labels
labels = kmeans.labels_
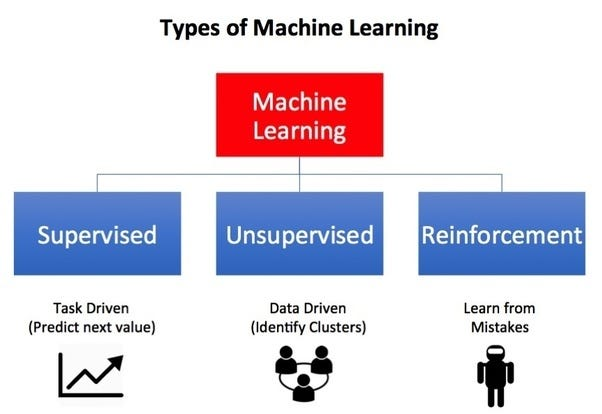
Reinforcement Learning
Reinforcement learning is a type of machine learning where an agent learns to take actions in an environment to maximize a reward signal. The agent learns through trial and error, receiving rewards or penalties for its actions.
# Reinforcement learning example using Q-learning
import numpy as np
# Defining the environment
env = np.array([[0, 0, 0, 0],
[0, 1, 0, 0],
[0, 0, 0, 1],
[0, 0, 0, 0]])
# Defining the Q-learning algorithm
q_table = np.zeros((env.shape[0], env.shape[1]))
# Learning the Q-table
for episode in range(1000):
state = 0
done = False
rewards = 0
while not done:
action = np.random.randint(0, env.shape[1])
reward = env[state, action]
q_table[state, action] = (1 - 0.5) * q_table[state, action] + 0.5 * (reward + 0.5 * np.max(q_table[state, :]))
state = action
rewards += reward
if reward == 1:
done = True
print(f'Episode {episode+1}, Reward: {rewards}')
Applications of Machine Learning
Machine learning has numerous applications across industries, including:
Healthcare
Machine learning is revolutionizing healthcare by improving diagnosis accuracy, streamlining clinical workflows, and enabling personalized medicine.
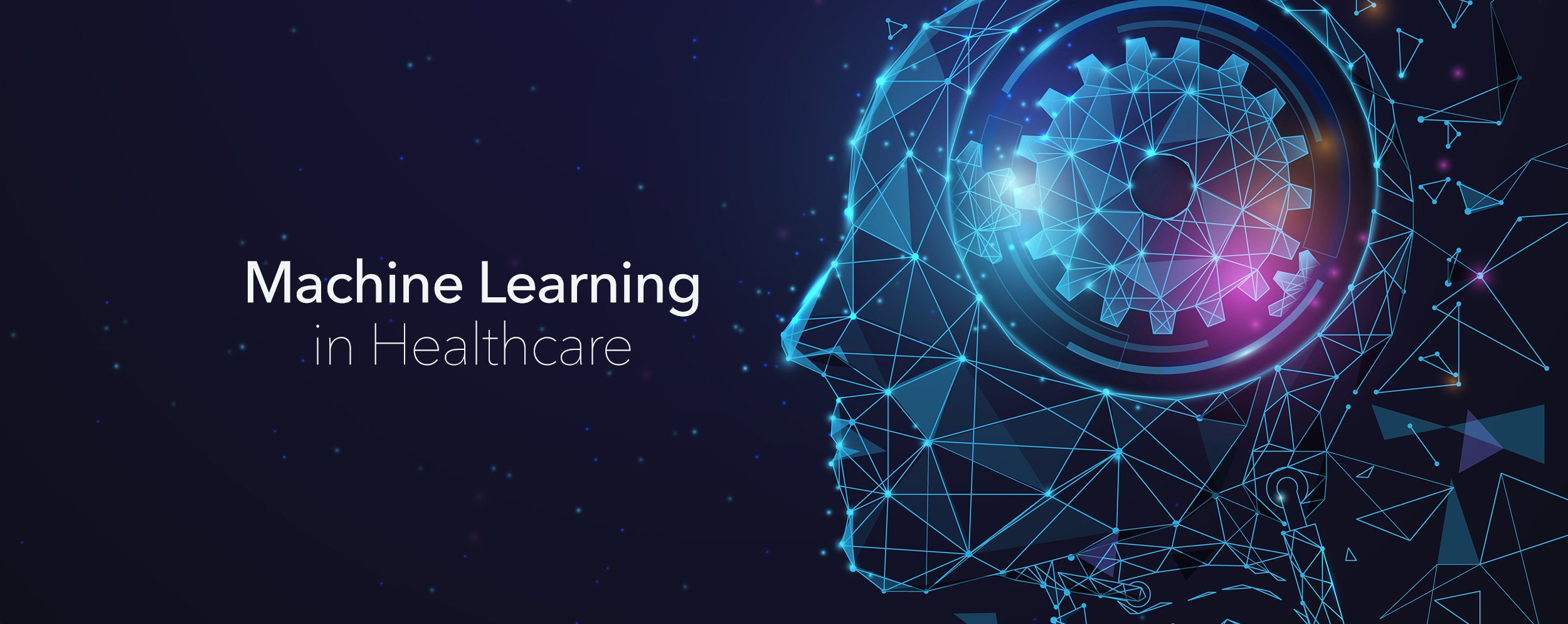
Finance
Machine learning is being used in finance to detect fraud, predict stock prices, and optimize investment portfolios.
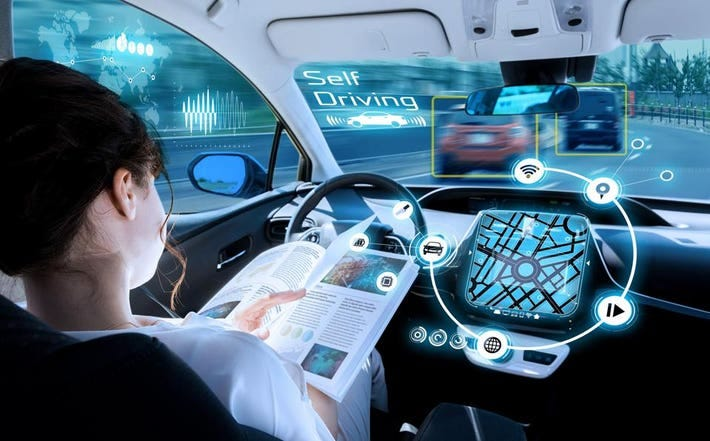
Transportation
Machine learning is powering the development of self-driving cars, trucks, and drones, revolutionizing the transportation industry.
# Recommendation system example using scikit-surprise
from surprise import KNNWithMeans
from surprise import Dataset
from surprise.model_selection import train_test_split
# Loading the movie lens dataset
data = Dataset.load_builtin('ml-100k')
# Splitting the data into training and testing sets
trainset, testset = train_test_split(data, test_size=.25)
# Training the recommendation model
algo = KNNWithMeans(k=50, sim_options={'name': 'pearson', 'user_based': False})
algo.fit(trainset)
# Making recommendations
predictions = algo.test(testset)
Conclusion
Machine learning is a powerful technology with far-reaching applications across industries. From healthcare to finance, transportation to education, machine learning is revolutionizing the way we live and work. As the volume and complexity of data continue to grow, the demand for skilled machine learning practitioners will increase. Whether you're a seasoned developer or a curious beginner, now is the perfect time to dive into the world of machine learning and unlock its immense potential.
Start Building Your Machine Learning Project Today!
- Machine Learning by Andrew Ng on Coursera](https://www.coursera.org/specializations/machine-learning)
- Machine Learning with Python by Sci-kit learn
- Start building your machine learning project with Python and scikit-learn today!